Bayesian finance: Predicting financial outcomes with sustainability data
Leveraging ESG and financial data for better predictions and causal insights
TLDR: Bayesian statistics can be applied to financial modeling, with a focus on integrating sustainability metrics (like ESG factors) alongside traditional financial data. By using Bayesian inference, we can continually update our predictions on variables like share prices and profits as new data becomes available. We’ll also dive into how this approach captures causal relationships between sustainability efforts and financial performance, providing deeper insights for investment strategies. For financial professionals, Bayesian methods offer a powerful, forward-thinking tool to enhance decision-making in today’s complex, data-rich markets.
Please note that this is our first paywalled article. Free articles still await you every Tuesday. In our paywalled Friday editions, we will be digging deeper into the technical aspects of our work. Subscribe now to read the whole article, or reach out to us at contact@wangari.global if you need a student discount.
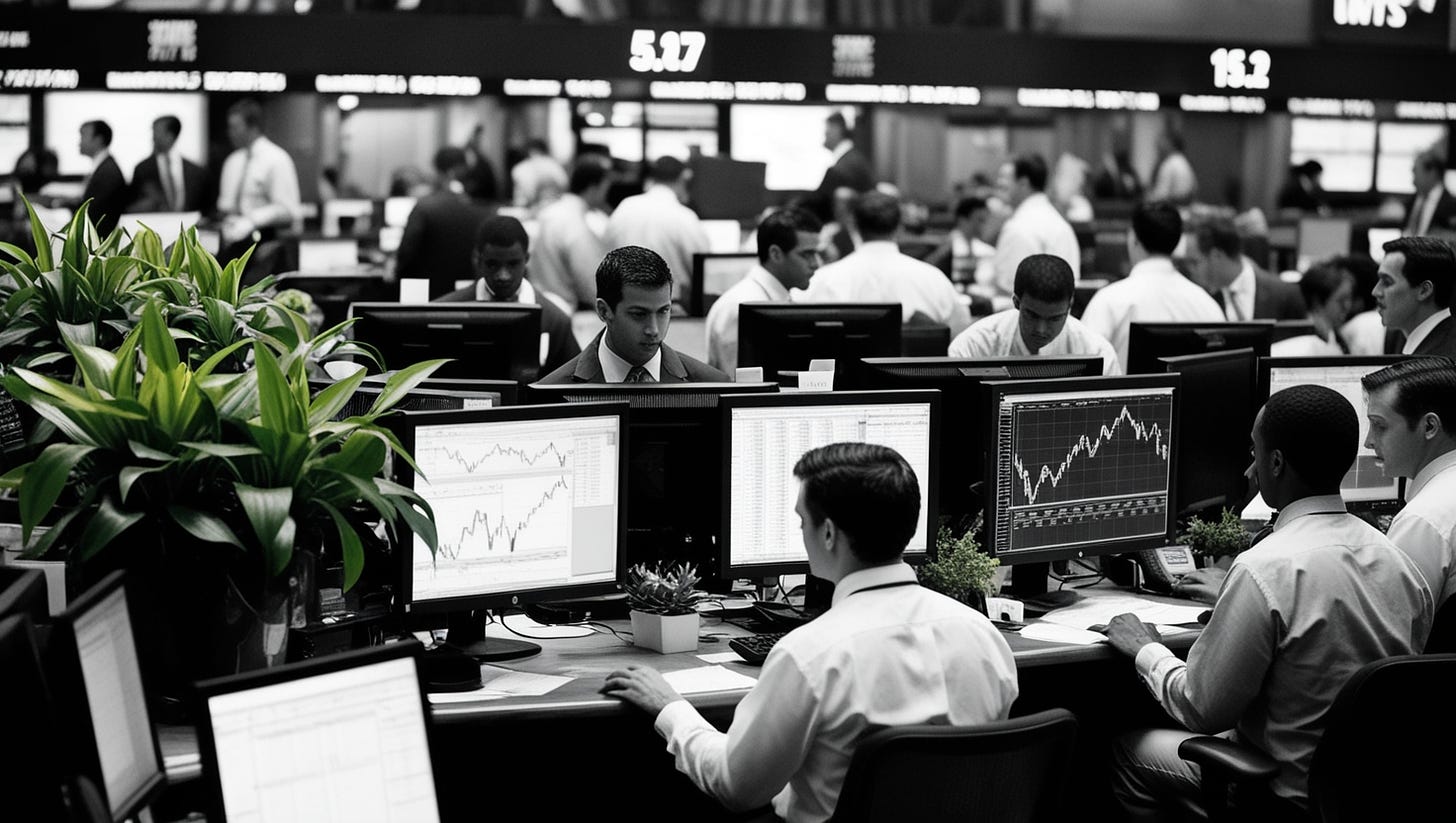
In today’s fast-evolving financial markets, traditional models often struggle to keep up with the complexity and uncertainty of real-world data. This is especially true when incorporating sustainability metrics—such as environmental, social, and governance (ESG) factors—alongside financial data. Investors and financial institutions are increasingly interested in understanding the impact of sustainability on performance, yet conventional models tend to treat these factors as static or secondary, failing to fully account for their dynamic influence over time.
Bayesian statistics offers a solution to this challenge by providing a framework that continuously updates predictions based on new information. Unlike classical statistical methods, which rely on fixed assumptions, Bayesian models allow us to incorporate prior beliefs—such as historical financial performance and sustainability efforts—and adjust these predictions as new data becomes available. This dynamic approach not only makes predictions more flexible but also more aligned with real-world conditions.
For financial professionals, the use of Bayesian inference opens up exciting possibilities. By combining sustainability metrics with traditional financial indicators, it becomes possible to make more informed predictions about future share prices, profitability, and even long-term risks. Bayesian approaches also offer the ability to infer causal relationships between sustainability initiatives and financial outcomes, enabling investors to develop more accurate, forward-looking strategies in an increasingly complex market environment.
Bayesian statistics explained: A primer
Bayesian statistics is built on the idea of updating beliefs in the face of new evidence. Named after the 18th-century statistician Thomas Bayes, this approach revolves around Bayes' theorem, which provides a mathematical framework for adjusting prior beliefs based on the likelihood of observed data.
In financial modeling, this flexibility makes Bayesian methods particularly powerful for dealing with uncertainty and the ever-changing nature of markets. In my past as a particle physics researcher, I have used Bayesian approaches to assess the likelihood of a certain particle (e.g. a dark matter particle or a Higgs boson) coming out of a collision of protons (as they occur most famously at CERN) or from cosmic sources. Whether financial markets or particle physics, the principle remains the same though.
At its core, Bayesian inference starts with a prior—an initial assumption or belief about a particular outcome. In the context of financial modeling, this could be a company’s expected profitability based on historical data or an assumption about how environmental practices will impact future performance. As new data becomes available, such as updated financial statements or sustainability reports, these priors are updated to form a posterior—a refined belief or prediction that better reflects the current reality.
The key components of Bayesian analysis in finance are therefore:
Prior distribution: This represents our initial beliefs about the parameters before observing any data. In finance, priors can incorporate expert knowledge, historical data, or theoretical models.
Likelihood function: This describes the probability of observing the data given the model parameters.
Posterior distribution: This is the updated belief about the parameters after observing the data, combining the prior and likelihood.
Unlike traditional statistical models, which often rely on fixed parameters, Bayesian models continuously adjust and become more accurate as more data is incorporated. For example, in asset pricing, Bayesian models can take into account not only past financial performance but also external factors like regulatory changes related to sustainability, thus improving the prediction of future share prices or profitability.
In particular, Bayesian models offer several benefits over more traditional frequentist approaches:
Incorporation of prior knowledge: Bayesian analysis allows for the integration of existing information or expert opinions into the model, which is particularly valuable in finance where historical data and market insights are crucial.
Uncertainty quantification: Bayesian methods provide a natural way to quantify uncertainty in parameter estimates and predictions, which is essential for risk management in finance.
Flexibility in model complexity: Bayesian approaches can handle complex, hierarchical models that are often necessary to capture the intricacies of financial systems.
Handling of small sample sizes: In finance, where rare events or limited data are common, Bayesian methods can provide more robust estimates compared to frequentist approaches.
In addition to their flexibility, Bayesian models also offer a clear way to quantify uncertainty, which is crucial for financial institutions. By integrating both sustainability-related and financial metrics into a unified model, Bayesian statistics provide a structured way to deal with the complexity of modern financial markets, offering insights that are more robust and reflective of real-world dynamics.
Defining priors: Sustainability metrics and financial metrics
In Bayesian statistics, priors represent initial assumptions or beliefs about an outcome before incorporating new data. In the context of financial modeling, choosing the right priors is critical to making accurate predictions. For financial institutions, these priors often consist of traditional financial metrics like earnings, debt levels, or market performance. However, with the increasing focus on sustainability, integrating sustainability metrics—such as environmental, social, and governance (ESG) factors—into priors provides a more holistic view of a company's future prospects.
Sustainability metrics are powerful indicators of long-term value creation or risk exposure. For instance, a company’s carbon footprint, governance structure, or social impact initiatives can affect its regulatory risks, reputation, and even profitability. Forward-thinking investors are increasingly using these ESG factors as part of their investment decision-making process. In a Bayesian framework, these metrics serve as priors, helping to inform future expectations and outcomes for a company’s stock price or profitability.
Consider a company with a strong track record of environmental responsibility, indicated by its low emissions and active participation in sustainability initiatives. By using these ESG metrics as priors, Bayesian models can adjust predictions of financial performance to account for the potential benefits of this company’s sustainable practices—whether through lower regulatory risks, reduced operational costs, or enhanced market reputation.
On the financial side, traditional metrics such as revenue growth, earnings per share (EPS), and price-to-earnings (P/E) ratios remain essential. These provide a baseline for understanding a company’s past performance and are crucial to setting realistic prior expectations. However, by integrating both financial and sustainability metrics into a Bayesian model, we can develop a more comprehensive view. For example, a company with both strong financial fundamentals and robust sustainability practices might have a lower risk profile and a higher probability of delivering long-term returns.
In practice, selecting priors in Bayesian models is an art as much as a science. The choice of priors must reflect the best available data and expert judgment. By combining sustainability and financial data, financial institutions can make predictions that are not only data-driven but also aligned with broader trends in sustainable finance, providing a more accurate and nuanced outlook on investment outcomes.
Posterior predictions: From share prices to profits
Once priors are established—whether they are based on sustainability metrics, financial performance, or a combination of both—Bayesian inference moves toward calculating the posterior. This is where the true power of Bayesian statistics emerges: it updates predictions as new data becomes available, leading to a refined understanding of future outcomes such as share prices, profitability, or other financial indicators.
In a financial context, the posterior is the adjusted prediction after incorporating the latest available data. For instance, if a company with strong ESG metrics suddenly faces regulatory changes due to new environmental laws, a Bayesian model would adjust the predicted stock price or profitability based on this new information. The posterior represents this updated belief about the company’s future performance.
Keep reading with a 7-day free trial
Subscribe to Wangari Digest to keep reading this post and get 7 days of free access to the full post archives.